Vignette¶
Exemplary analysis of the PBMC3K single-cell RNA dataset.
[2]:
import os
import math
import warnings
import matplotlib.pyplot as plt
import pandas as pd
import scanpy as sc
from keggtools import (
Pathway,
Enrichment,
EnrichmentResult,
Resolver,
Renderer,
IMMUNE_SYSTEM_PATHWAYS,
)
from IPython.display import Image, display
# Used folders
figure_dir = os.path.join(os.getcwd(), "figures")
# Global settings
sc.settings.verbosity = 0
sc.logging.print_header()
sc.settings.set_figure_params(dpi=80, facecolor="white")
# Ignore all warnings
warnings.filterwarnings("ignore", category=UserWarning)
scanpy==1.10.4 anndata==0.11.1 umap==0.5.1 numpy==2.0.2 scipy==1.14.1 pandas==2.2.3 scikit-learn==1.6.0 statsmodels==0.14.4 igraph==0.11.8 pynndescent==0.5.13
Load preprocessed PMBC3K dataset¶
[3]:
adata = sc.datasets.pbmc3k_processed()
Plot UMAP with cluster annotations¶
[24]:
# Plot and save UMAP
fig, ax = plt.subplots(1, 1, figsize=(10, 8))
ax.set_aspect("equal", adjustable="box")
sc.pl.umap(adata, color="louvain", legend_loc="right margin", title="", frameon=True, ax=ax, show=False)
fig.tight_layout(pad=3.0)
fig.savefig(os.path.join(figure_dir, "figure2.png"))
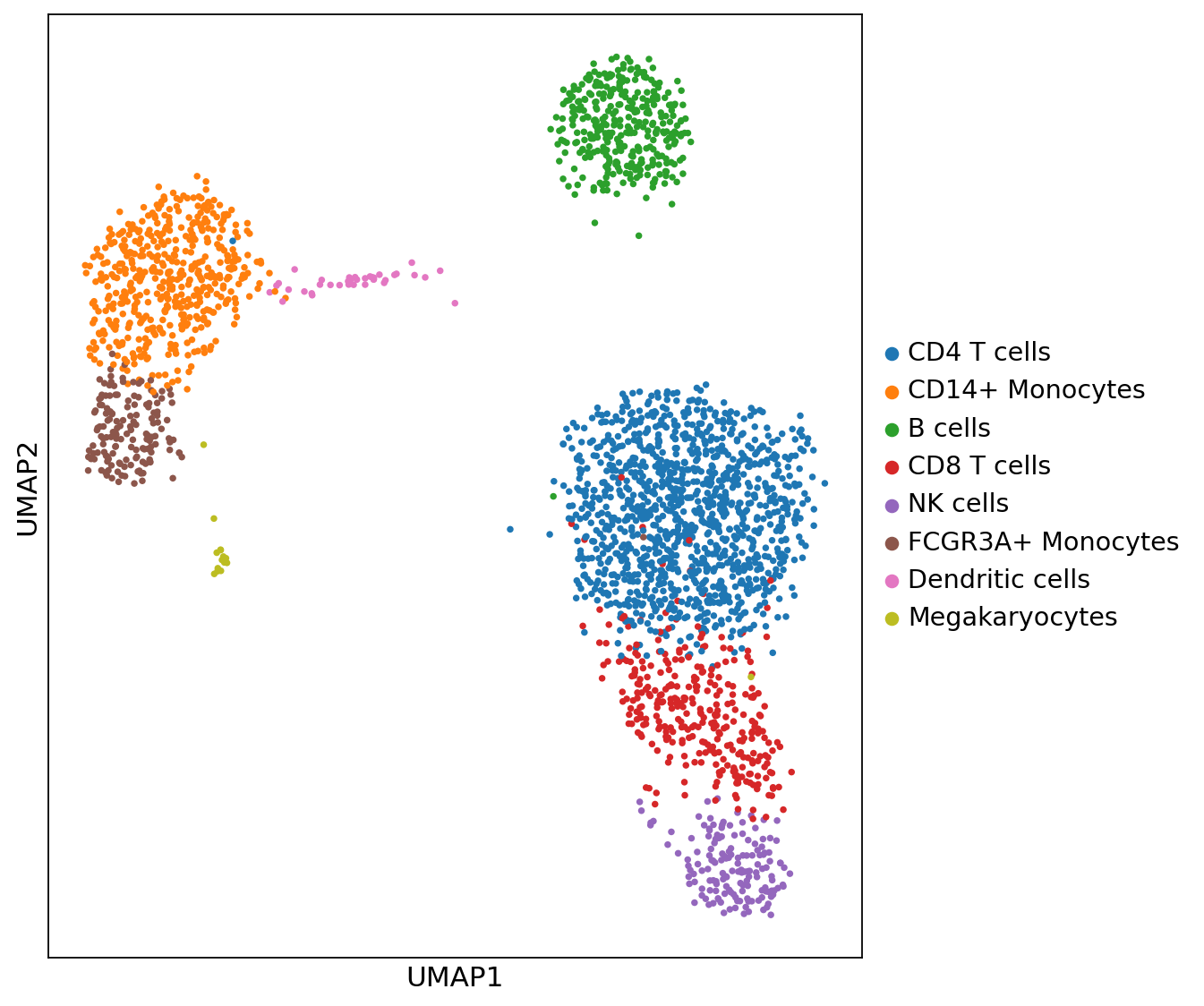
Differential analysis results to pandas dataframe¶
[5]:
sc.tl.rank_genes_groups(adata, "louvain", method="wilcoxon", n_genes=100)
[6]:
# Export diff exp data
diffexp_df = sc.get.rank_genes_groups_df(adata, group="NK cells", pval_cutoff=0.05, log2fc_min=1)
diffexp_df.head()
[6]:
names | scores | logfoldchanges | pvals | pvals_adj | |
---|---|---|---|---|---|
0 | NKG7 | 20.181747 | 5.623642 | 1.416465e-90 | 1.942540e-86 |
1 | GZMB | 19.738880 | 6.650631 | 9.996947e-87 | 6.854906e-83 |
2 | PRF1 | 19.434803 | 5.433477 | 3.919145e-84 | 1.791572e-80 |
3 | GNLY | 19.357994 | 6.657686 | 1.745530e-83 | 5.984549e-80 |
4 | CTSW | 18.578722 | 3.808060 | 4.777493e-77 | 1.310371e-73 |
Perform KEGGTOOLS enrichment analysis¶
[7]:
organism_id: str = "hsa"
[8]:
from keggtools.utils import merge_entrez_geneid
[9]:
diffexp_df = merge_entrez_geneid(diffexp_df)
diffexp_df.head()
[9]:
names | scores | logfoldchanges | pvals | pvals_adj | symbol | entrez | |
---|---|---|---|---|---|---|---|
0 | NKG7 | 20.181747 | 5.623642 | 1.416465e-90 | 1.942540e-86 | NKG7 | 4818 |
1 | GZMB | 19.738880 | 6.650631 | 9.996947e-87 | 6.854906e-83 | GZMB | 3002 |
2 | PRF1 | 19.434803 | 5.433477 | 3.919145e-84 | 1.791572e-80 | PRF1 | 5551 |
3 | GNLY | 19.357994 | 6.657686 | 1.745530e-83 | 5.984549e-80 | GNLY | 10578 |
4 | CTSW | 18.578722 | 3.808060 | 4.777493e-77 | 1.310371e-73 | CTSW | 1521 |
[10]:
# Init resolver instance
resolver: Resolver = Resolver()
pathway_list: list[Pathway] = []
# Download all immune system pathways
for number, _ in IMMUNE_SYSTEM_PATHWAYS.items():
pathway_list.append(resolver.get_pathway(organism=organism_id, code=number))
[11]:
# Init enrichment from list of immune system pathways
analysis: Enrichment = Enrichment(pathways=pathway_list)
analysis_result: list[EnrichmentResult] = analysis.run_analysis(gene_list=list(diffexp_df["entrez"]))
result_df: pd.DataFrame = analysis.to_dataframe()
# Filter out pathways with no genes found
result_df = result_df[result_df["study_count"] > 0]
# Print out result
result_df.head()
[11]:
pathway_name | pathway_title | pathway_id | study_count | pathway_genes | pvalue | found_genes | |
---|---|---|---|---|---|---|---|
0 | path:hsa04640 | Hematopoietic cell lineage | 04640 | 1 | 100 | 0.183444 | 924 |
1 | path:hsa04610 | Complement and coagulation cascades | 04610 | 1 | 88 | 0.259797 | 3689 |
2 | path:hsa04611 | Platelet activation | 04611 | 2 | 126 | 0.235741 | 5908,2207 |
3 | path:hsa04620 | Toll-like receptor signaling pathway | 04620 | 3 | 109 | 0.799511 | 6352,6348,6351 |
4 | path:hsa04621 | NOD-like receptor signaling pathway | 04621 | 1 | 189 | 0.005539 | 6352 |
Plot results of enrichment analysis¶
[23]:
plt.figure(figsize=(8, 5), dpi=300)
scatter = plt.scatter(
x=result_df["study_count"] / result_df["pathway_genes"] * 100,
y=result_df["pathway_title"],
c=[-math.log10(x) for x in result_df["pvalue"]],
cmap="coolwarm",
)
cbar = plt.colorbar()
cbar.set_label("- log10(p value)")
plt.grid(visible=None)
plt.tight_layout()
plt.savefig(os.path.join(figure_dir, "figure4.png"), bbox_inches="tight")
plt.show()
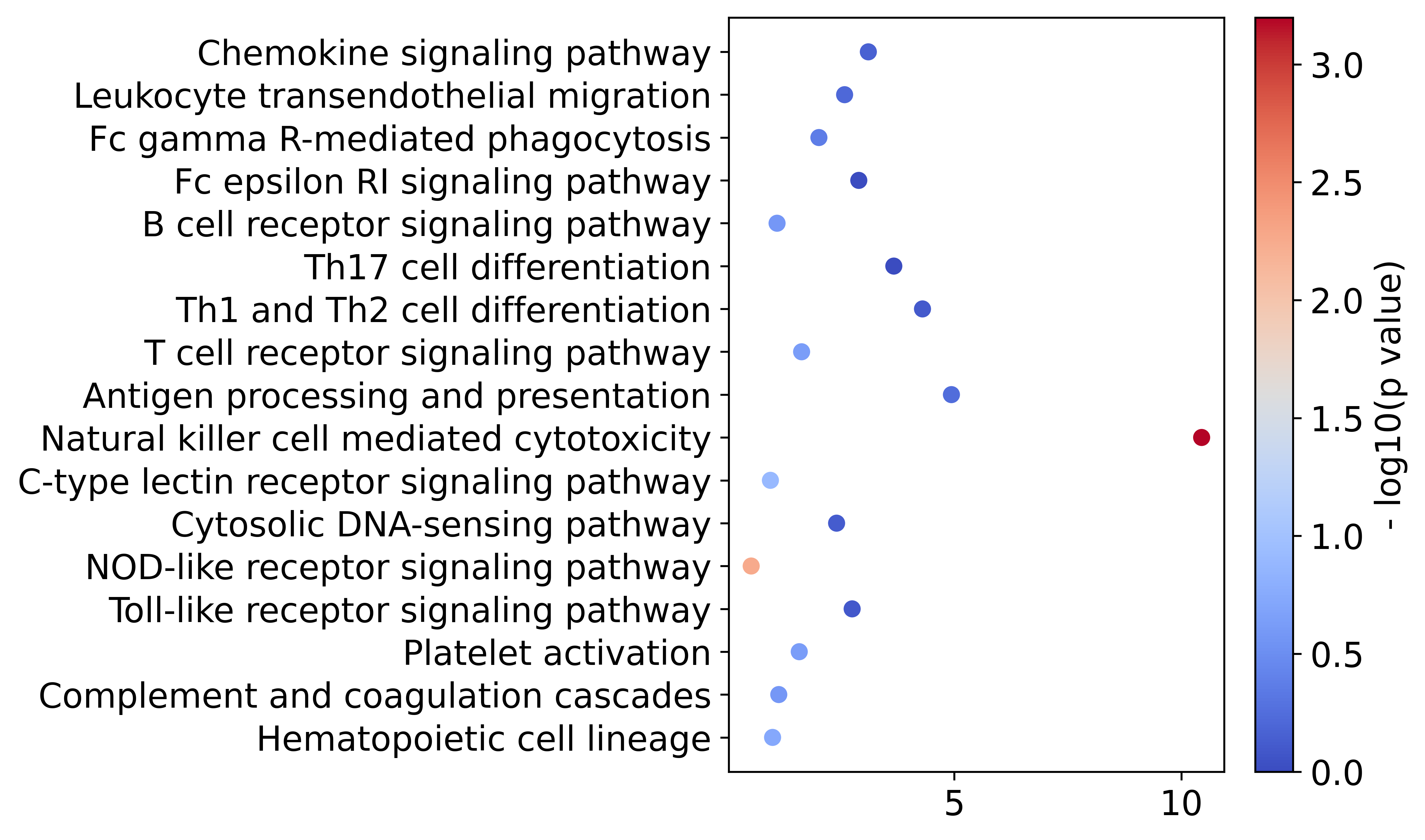
Plot pathway¶
“Antigen processing and presentation” (hsa:04612) show a significant p value
[13]:
pathway: Pathway = resolver.get_pathway(organism=organism_id, code="04650")
[14]:
# diffexp_df[["entrez"]] = diffexp_df[["entrez"]].astype(int)
overlay: dict = dict(zip(list(diffexp_df["entrez"]), list(diffexp_df["logfoldchanges"]), strict=True))
[15]:
renderer: Renderer = Renderer(kegg_pathway=pathway, gene_dict=overlay, cache_or_resolver=resolver.storage)
renderer.render(display_unlabeled_genes=True)
[16]:
# Save dot string to file
with open(os.path.join(figure_dir, "figure5.dot"), "w") as file_obj:
file_obj.write(renderer.to_string())
# Save binary data to file
renderer.to_file(filename=os.path.join(figure_dir, "figure5.png"), extension="png")
[17]:
# Display image
img: Image = Image(os.path.join(figure_dir, "figure5.png"))
display(img)
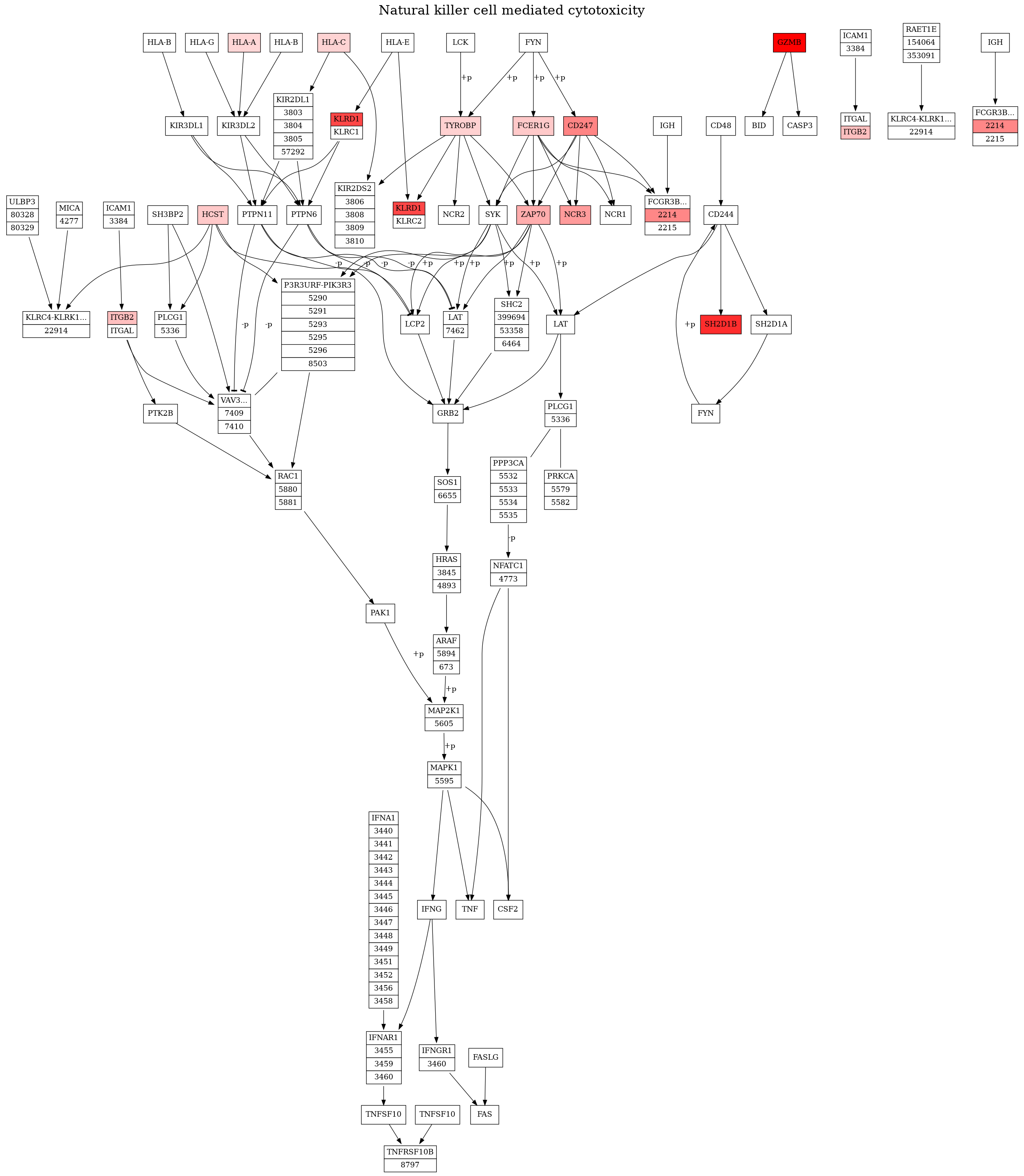